MMInstruct: A High-Quality Multi-Modal Instruction Tuning Dataset with Extensive Diversity
Jul 22, 2024ยท
,,,,,,,,,,ยท
1 min read
Yangzhou Liu

Yue Cao
Zhangwei Gao
Weiyun Wang
Zhe Chen
Wenhai Wang
Hao Tian
Lewei Lu
Xizhou Zhu
Tong Lu
Yu Qiao
Jifeng Dai
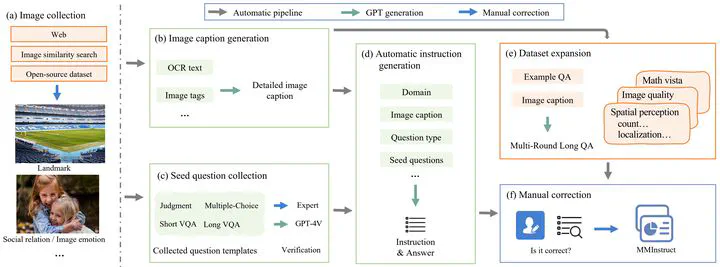
Abstract
Despite the effectiveness of vision-language supervised fine-tuning in enhancing the performance of Vision Large Language Models (VLLMs). However, existing visual instruction tuning datasets include the following limitations: (1) Instruction annotation quality: despite existing VLLMs exhibiting strong performance, instructions generated by those advanced VLLMs may still suffer from inaccuracies, such as hallucinations. (2) Instructions and image diversity: the limited range of instruction types and the lack of diversity in image data may impact the model’s ability to generate diversified and closer to real-world scenarios outputs. To address these challenges, we construct a high-quality, diverse visual instruction tuning dataset MMInstruct, which consists of 973K instructions from 24 domains. There are four instruction types: Judgement, Multiple-Choice, Long Visual Question Answering and Short Visual Question Answering. To construct MMInstruct, we propose an instruction generation data engine that leverages GPT-4V, GPT-3.5, and manual correction. Our instruction generation engine enables semi-automatic, low-cost, and multi-domain instruction generation at 1/6 the cost of manual construction. Through extensive experiment validation and ablation experiments, we demonstrate that MMInstruct could significantly improve the performance of VLLMs, e.g., the model fine-tuning on MMInstruct achieves new state-of-the-art performance on 10 out of 12 benchmarks. The code and data shall be available at https://github.com/yuecao0119/MMInstruct.
Type
Publication
SCIENCE CHINA Information Sciences
Citation
If you find this project useful in your research, please consider cite:
@article{liu2024mminstruct,
title={Mminstruct: A high-quality multi-modal instruction tuning dataset with extensive diversity},
author={Liu, Yangzhou and Cao, Yue and Gao, Zhangwei and Wang, Weiyun and Chen, Zhe and Wang, Wenhai and Tian, Hao and Lu, Lewei and Zhu, Xizhou and Lu, Tong and others},
journal={Science China Information Sciences},
volume={67},
number={12},
pages={1--16},
year={2024},
publisher={Springer}
}